Introduction to Statistical Inference
Statistical inference is the process of using data analysis to make inferences about a population or process that go beyond what is already known. By testing hypotheses and calculating estimates, inferential statistical analysis infers population properties. For example, you may survey a sample of individuals in a region and make specific inferences based on that sample utilizing statistical principles such as simulation and probability theory.
This is one of the best online Statistical Inference courses. You will learn about modern statistical concepts and processes that are drawn from a mathematical foundation in this course. This course is intended for advanced undergraduates, statistics master's students, and STEM and other doctoral candidates. You will gain a thorough understanding of how statistics function, which will prepare you for further statistics coursework. Some computations in the R programming language may be required for assignments. A Bachelor's degree conferred with a 3.3 or above undergraduate GPA.
What you will learn
- The ideas that underpin statistical approaches, such as sample vs. population, are discussed.
- Testing, estimation, confidence intervals, and model selection are examples of inferential tasks.
- How to apply models based on a few different types of distributions, such as normal, binomial, and Poisson
Prerequisites - Basic combinatorics, discrete and continuous random variables, special distributions, laws of large numbers, and the central limit theorem are all covered in this rigorous introductory probability course. Advanced calculus: understanding of infinite series, limits, and double integrals.
This course include
- Decision theory
- Point and interval estimation
- Tests of hypotheses
- Neyman-Pearson theory
- Bayesian analysis
- Maximum likelihood
- Large sample theory
Offered by: Stanford University
Enroll here: https://online.stanford.edu/courses/stats200-introduction-statistical-inference
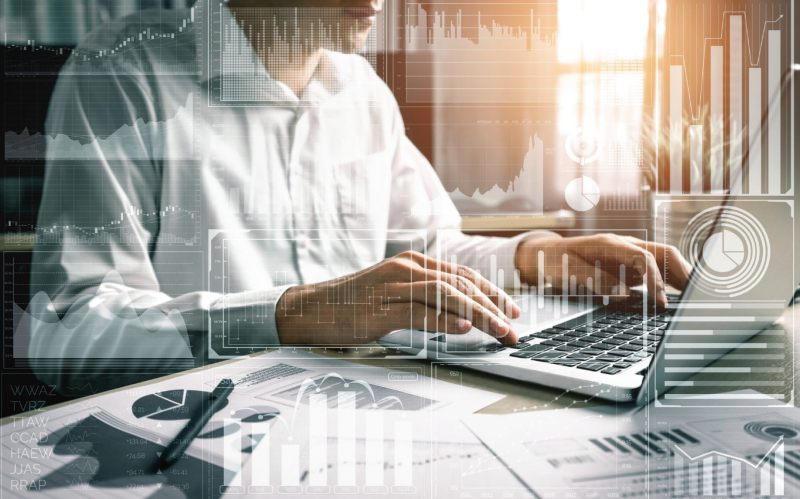
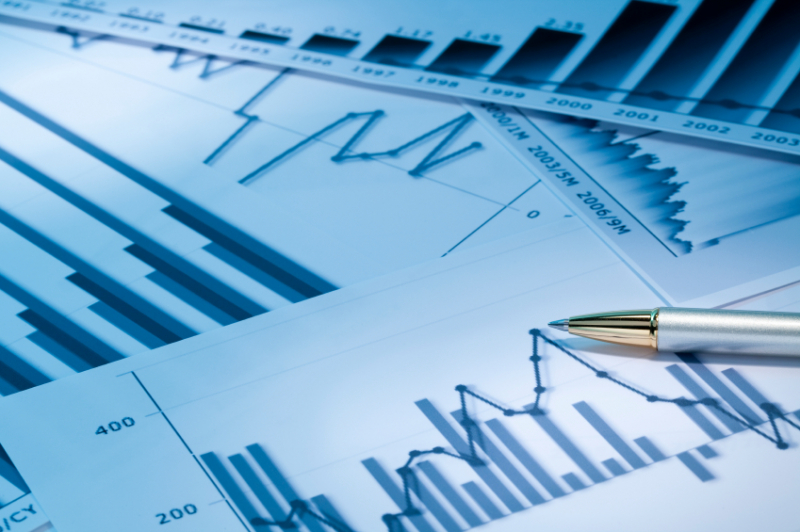