Statistical Inference and Hypothesis Testing in Data Science Applications
This course will focus on the theory and application of hypothesis testing, particularly as it relates to data science applications and statistical inference. Students will be taught how to use hypothesis testing to create data-driven decisions. The general logic of hypothesis testing, error and error rates, power, simulation, and the correct computation and interpretation of p-values will be given special focus. Usage of testing ideas, particularly p-values, will be discussed, as well as the ethical implications of such misuse.
This course is part of CU Boulder's Master of Science in Data Science (MS-DS) program, which is available on the Coursera platform. The MS-DS is an interdisciplinary degree that brings together faculty from the Applied Mathematics, Computer Science, Information Science, and other departments at CU Boulder. The MS-DS is excellent for persons with a broad variety of undergraduate education and/or professional experience in computer science, information science, mathematics, and statistics, as it is based on performance rather than application.
What you will learn
- For a test with a composite null hypothesis, define a composite hypothesis and the degree of significance.
- Define a hypothesis test's test statistic, level of significance, and rejection region. Create a rejection region in the form of a rectangle.
- Conduct tests to determine the genuine population variance.
- Calculate the exponential distribution's sampling distributions for the sample mean and sample minimum.
Instructor: Jem Corcoran
Coursera rate: N/A
Offered by: University of Colorado Boulder
Enroll here: https://www.coursera.org/learn/statistical-inference-and-hypothesis-testing-in-data-science-applications
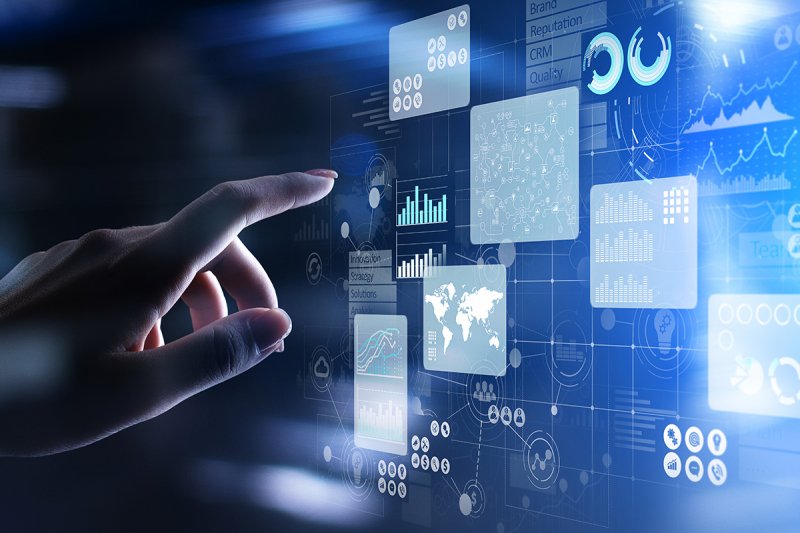
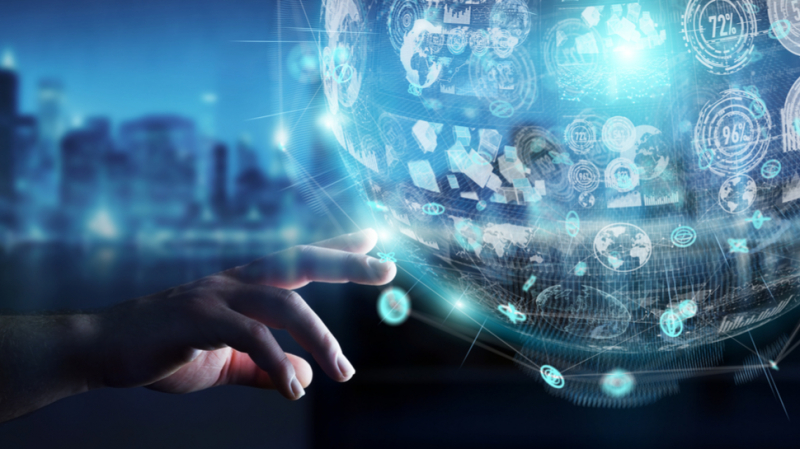